Data science can have incredible benefits for your business. However, it’s important to understand that it’s a solution to a problem, not a way to find the problem. It means that if your company has a lot of data that you don’t quite know what to do with, you need to figure out what you are trying to improve or change before hiring a team of data scientists. On this point, data science consulting can help you. Data scientists analyze data to find insights, but it’s the job of product managers and business leaders to tell them what to look for.
There are multiple ways of how to use data science in business. If you are in the process of figuring out exactly what benefits of data science for business have value to your company, you can consider the following ways of using data science:
- building better products
- making better decisions
- automating repetitive, time-consuming processes
Let’s take a closer look at these three areas.
Using Data Science to Build Better Products
By using data science in business, you can bring a better product to your target market in two main ways: you can either customize a product or service to make it more personal or you can provide a new experience with the product or service.
Today machine learning looks most attractive for businesses in terms of generating real value and enabling breakthrough innovation. There are three main types of machine learning algorithms: unsupervised, supervised, and reinforcement learning. We are going to focus on the first two and share with you real-world examples of how these algorithms can benefit your product.
The discussion about supervised and unsupervised learning can get complex and technical at times. Essentially, supervised learning is about predicting an outcome, while unsupervised learning is about identifying a pattern. Both of them can help you deliver better products to your customers by understanding them better.
Unsupervised learning allows you to capture your customer preferences and use the data to anticipate their needs and behaviors in the future. The most common examples of unsupervised learning are Amazon’s recommendations based on what other customers also bought as well as Spotify’s suggestions for your playlist based on the songs you’ve already liked or added. To build this type of recommendations, data scientists solve a clustering problem, grouping similar users together to form homogeneous clusters.
Supervised learning is used in predicting customer behavior. By solving a classification problem, machine learning engineers may help you identify satisfied and unsatisfied customers and predict churn. By solving a recommendation problem, data scientists try to guess things that your customers may be interested in. By solving a ranking problem, data scientists help users find the right thing faster when they search.
Supervised learning is also used to enable features such as face recognition, image classification, speech recognition. These features revolutionize customer experience and make tech products more intuitive to use, like telling virtual assistants to schedule a meeting instead of accessing scheduling software to find a time, create an event, and type the details.
The great thing about machine learning is that it is especially helpful in optimizing user engagement and retention, both of which are critical success factors for your product. Share on XImportance of Data Science to Make Better Decisions
With data science and predictive analytics, in particular, you can predict useful metrics and trends for your business. Such an approach can help you improve your ability to serve your customers or otherwise compete in the market. The importance of data science and predictive analytics, i.e., in the finance industry, is in the fact that organizations can leverage the power of technology to detect what can negatively impact businesses before these issues happen or spread.
Predictive analytics is not a new field, but where and how you can apply it has grown thanks to recent advancements in technology. Today predictive analytics is all about connecting disparate systems and data sets to do proper analysis and derive valuable insight out of seemingly chaotic data.
Solutions powered by advanced analytics have a big potential to reduce costs that arise due to failures, bottlenecks, customer churn, etc. A good example of predictive analytics in action is anomaly detection for IoT by Anodot. By using machine learning algorithms, Anadot analytics platform keeps machines running smoothly by flagging anomalies in the data. If a machine is starting to show signs that it needs maintenance or repair, algorithms can understand this from small changes in the sensor data. Such proactive maintenance can keep support costs down and customers happy.
Advanced analytics introduces the ability to leverage the power of multiple data sets and discover connections where none could have been found before. A good example of this is when the New York City government, in 2016, was trying to reduce the costs related to legal claims against the city. By putting together the data from all departments and applying advanced analytics, the city found correlations that were not obvious to the human eye. One of them was that the amount of tree-related accidents spiked after a major budget cut was introduced in the Parks and Recreations department.
With the increase in accumulated data (IBM predicted a 42% increase by 2020), advanced analytics will become the norm rather than a way to gain competitive advantage. Here is the list of helpful tips on the uses of data science in business and implementation of advanced analytics:
Five steps to implement effective predictive analytics solutions
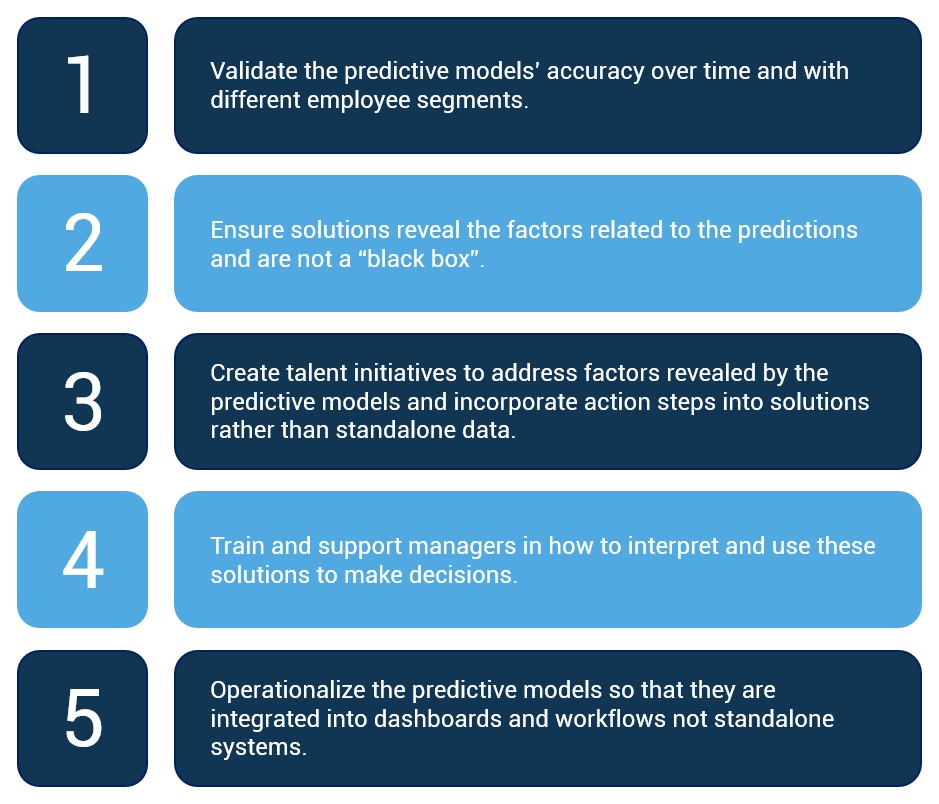
At InData Labs, we have a wealth of experience, proved by client feedback, in building predictive analytics solutions. Our machine learning engineers use proprietary technologies to train models helpful in analyzing large datasets and extracting value from data.
So, for instance, our team cooperated with a business in the banking industry to help implement a machine learning model capable of improving decision making throughout the whole lifecycle. The client’s agency was engaged in debt collection, and InData Labs needed to explore the potential of predictive analytics for identifying the users most likely to repay.
Long story short, the model by InData Labs was able to predict the probability of promise to pay, which resulted in improved collection rates, optimized time of agency employees, and reduced operating costs. And to learn about building predictive models in detail, follow the link to a customer success story.
Using Data Science to Automate Processes
Automation is one of the hottest trends in modern technology. So, let’s discuss applications of data science in business for creating automated innovations.
To identify growth opportunities that automation can bring to your company, you can start by asking yourself:
- Where do people in my company spend a lot of time to make decisions that could be automated, so their skills could be better leveraged elsewhere?
- What types of data do people in my company normally search for and collect manually, and how can this be automated?
- Which tasks in my company can machines perform faster and more efficiently than humans?
Machine learning can free up resources through automatic retrieval, generation, or processing of content. It is becoming increasingly important in the age of large information repositories where the data contained does not possess any natural order.
For example, brand managers analyze large collections of images and posts from social media every day trying to figure out how, when, and where people use their product and how their customers feel about the brand. A social media analytics company Brandwatch uses machine learning to automate the process of image detection and analysis. Their product Image Insight helps collect and analyze more images containing your brand. That saves valuable time of your best people for where it actually matters.
More and more interactions take place through email, chat, and other e-channels. So, there is a chance to automate the tasks related to document retrieval, summarization, and classification. AI-powered text analysis is already used in legal discovery, helping to find all the documents that are relevant to a legal case. Searching through millions of emails and PDFs, looking for specific names and key phrases, is difficult for humans to do, but it’s pretty easy for a cluster of Elasticsearch nodes.
Decisions that normally require a high level of skills and knowledge — such as pricing, loan decisions, risk assessment and so on — can also be handed over to machine learning algorithms, provided that your data is clean and flows well between systems. That enables a quicker decision-making process, and the best part is that the model can learn from the outcomes and get better and better with time. However, this differs from full automation because such models will still have special cases and exceptions that need special review by the human experts from the field.
I hope the examples above have proved the importance of data science in business. That should give you food for thought on more practical innovations that you can implement within your organization with the help of data science. We’re looking forward to seeing more businesses successfully using machine learning in 2019.
Good luck out there!
Work with InData Labs on Your Data Science Project
Schedule an intro call with our Data Science consultants to explore your idea and find out if we can help.