In 2025, the integration of artificial intelligence (AI) and Big data analytics is poised to reshape industries, drive innovation, and enhance decision-making processes across various sectors.
Artificial intelligence stands at the forefront of global innovation as a beacon of certainty and data excellence. According to Markets and Markets, AI market size is expected to reach $407 billion by 2027.
Artificial intelligence market overview
The adoption of artificial intelligence has accelerated significantly in recent years, driven by advancements in technology, increased investment, and a growing recognition of AI’s transformative potential across various industries.
Here’s an overview of this remarkable growth, supported by key statistics.
Today: Main drivers of AI and Big data analytics
The global big data analytics market is projected to leap from $348.21 billion in 2024 to over $924 billion by 2032.
Big data and artificial intelligence have a synergetic relationship. To learn and enhance decision-making processes, AI needs a vast amount of data, and Big data analytics uses AI to improve data analysis. With this convergence, companies can swiftly glean insights from large stockpiles of data and more readily use sophisticated analytics capabilities like predictive analytics.
Strategies for AI adoption in 2025
In 2025, AI is evolving rapidly, creating new opportunities across industries. Successful adoption requires strategic planning, alignment with business goals, and choosing between custom AI for flexibility or off-the-shelf AI for quick, cost-effective implementation to maximize impact.
Advantages of the development of custom AI solutions:
- Tailored to specific needs: Custom models can be designed to meet the exact requirements of an organization’s problem or use case, leading to highly specialized solutions.
- Competitive advantage: Unique models can provide a competitive edge, as they are designed for specific business needs and are not available to competitors.
- Data privacy and security: Custom models can ensure that sensitive or proprietary data stays within the organization, which can be particularly important for sectors like healthcare and finance.
- Scalability: As the organization’s needs evolve, custom models can be adjusted and scaled to handle growing data or more complex tasks.
Disadvantages:
- Higher cost: Developing custom AI models often requires significant resources in terms of skilled personnel, computing power, and time. This can be expensive, especially for smaller organizations.
- Complexity: Building custom solutions involves handling data preprocessing, model development, and tuning, which can be complex and time-consuming.
- Skill requirements: Organizations must have the necessary technical expertise in-house to build and manage AI models, which can be a challenge for teams without experience in machine learning or data science.
Advantages of using off-the-shelf AI solutions
- Lower cost: Off-the-shelf solutions are generally more affordable because they don’t require the organization to develop the technology from scratch, and the cost is shared by multiple users.
- Quick deployment: Ready-made solutions can be implemented quickly, allowing organizations to start benefiting from AI technology without waiting for long development cycles.
- Ease of use: Many off-the-shelf tools are designed to be user-friendly and come with built-in support, making them accessible even to non-technical teams.
- Regular updates and support: Commercial AI solutions typically offer ongoing updates, bug fixes, and customer support, which can ease maintenance challenges.
Disadvantages:
- Limited customization: These solutions may not fully meet the specific needs of a business, leading to compromises in functionality or performance.
- Less competitive advantage: Since the solution is available to other companies, it doesn’t provide the same uniqueness or competitive edge as a custom-built model.
- Data privacy concerns: Using off-the-shelf solutions may involve sharing data with third-party providers, raising concerns about data security and privacy, especially in sensitive industries.
- Lack of flexibility: Some solutions might be rigid, making it difficult to adjust or scale the system as the organization’s needs evolve over time.
- Dependency on vendor: Organizations become reliant on the vendor for updates, support, and pricing, which can be risky if the vendor changes their business model or discontinues the product.
Custom AI models offer flexibility, control, and tailored solutions but come with high costs and maintenance requirements. Off-the-shelf solutions, on the other hand, are more cost-effective and easy to implement but may lack customization and can present data security concerns. The choice between the two depends on the organization’s specific needs, budget, and long-term goals.
The latest survey shows that off-the-shelf tools are widely applicable across industries, with approximately half of reported GenAI use cases involving these solutions with little or no customization. However, industries like energy, technology, media, and telecommunications are more likely to invest in significant customization or develop proprietary models to meet specific business needs.
Finance
In 2024, 91% of financials are either estimating or have already approved AI in their work. The AI in finance market size is projected to grow over $22.6 billion by 2026, exhibiting a 25.7% CAGR.
AI in finance helps to enhance work automation, establish more personalized service offerings and advance security risk management. The last one is the most effective and necessary in this area: 87% of businesses use AI adoption in financial services for fraud detection and anti-money laundering.
AI use cases for finance:
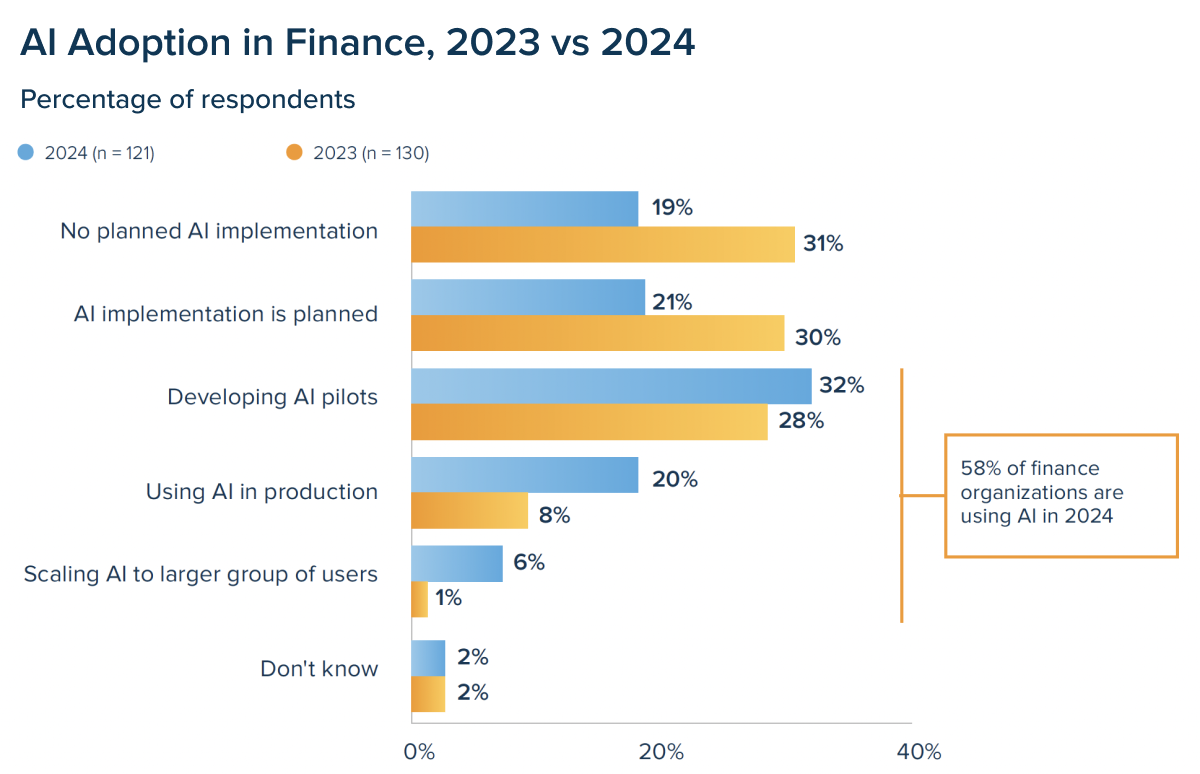
Healthcare
The healthcare industry has never been among early innovation adopters due to stringent regulations. However, AI in the healthcare market is projected to skyrocket to $194.4 billion by 2030.
The pursuit of digitalization and the COVID-19 pandemic strain have emphasized the need for proactive response and automation. As a result, organizations worldwide have started adopting AI models in the healthcare field. The biggest effect was shown in the areas of surgery and early diagnosis.
In 2023, 24.5% of the market was dominated by the robot-assisted surgery segment. It is also predicted that by 2025, 90% of hospitals will use AI for early diagnosis and remote monitoring.
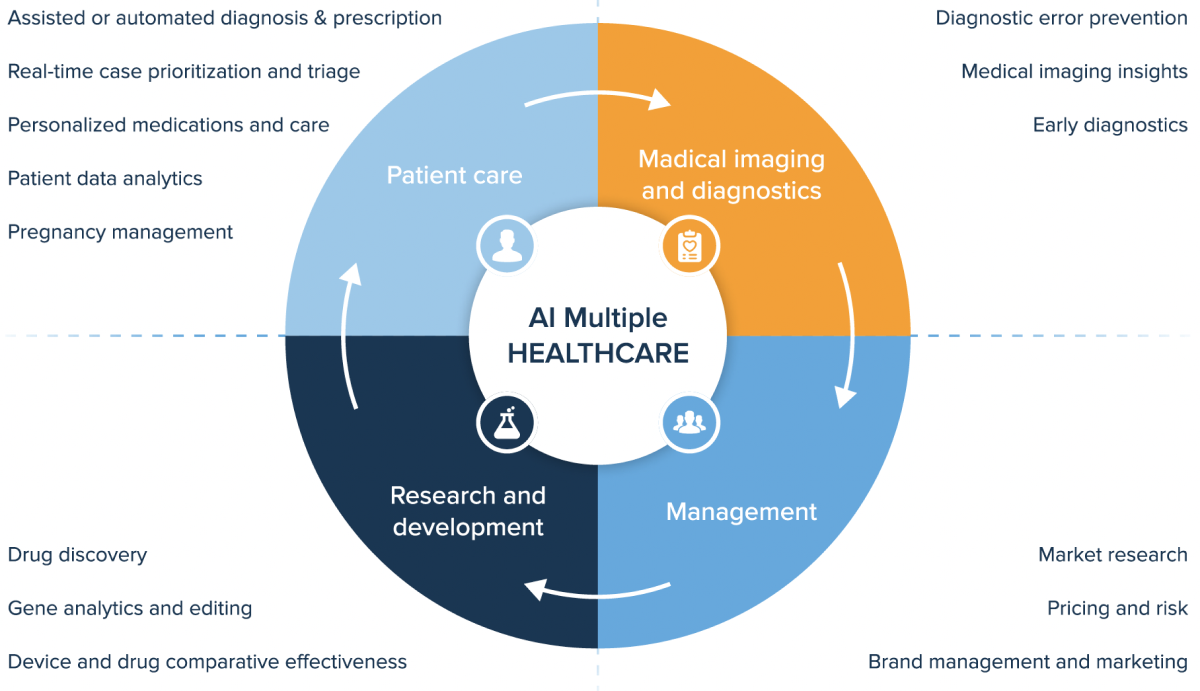
Manufacturing
The AI in manufacturing market size was USD 5.07 billion in 2023 and is forecasted to reach USD 68.36 billion by 2032, growing at a CAGR of 33.5% from 2023 to 2032.
Here are the top use cases for manufacturing:
This use case of AI for predictive maintenance empowers companies to observe equipment breakdowns proactively. It helps them minimize downtime and optimize maintenance schedules. By merging this digital twin with sensor data from actual machinery, AI in manufacturing can:
- Study patterns
- Spot anomalies
- Anticipate potential malfunctions, etc.
Retail
The global AI in retail market size is expected to grow at a CAGR of 23.0% from 2025 to 2030, starting from $11.61 billion in 2024.
Top AI retail use cases in 2025
Transformational technology: a Look ahead
Although AI and analytics are likely to orchestrate the majority of innovations, it remains difficult to predict the exact form and shape of intelligent transformation and plan ahead accordingly. We’ve curated the main technology trends to play out in the coming years with varying magnitude so that you can make strategic technology decisions.
Applied AI
Applied AI is the use of artificial intelligence to solve real-world problems. It involves the development of algorithms and models that can iteratively process and automatically learn from data to make predictions or decisions. Applied AI is different from general machine intelligence in that it is focused on specific tasks or problems such as increasing sales, reducing costs, or improving customer satisfaction rather than hypotheses.
The state of technology today:
Applied AI is the lifeblood of data analytics, statistics, machine learning, deep learning, artificial neural network, and NLP, with each having its wide application area across industries.
Advanced сonnectivity
Advanced connectivity refers to the various ways in which devices can connect and share data. It includes technologies like 5G, the Internet of Things, edge computing, wireless low-power networks, and other innovations that facilitate seamless and fast data sharing.
With an increasing number of devices, it is crucial to ensure connectivity to operate customer-centric markets, track supply chains, conduct proactive maintenance, and improve business processes.
The state of technology today:
The global IoT connectivity imperative has been driven by cellular IoT (2G, 3G, 4G, and now 5G) as well as LPWA over the last five years. Growing usage of medical IoT, IoT-enabled manufacturing, and autonomous vehicles have been among the greatest market enablers so far.
Web 3.0
Web 3.0 is the new iteration of the Internet that aims to make the digital space more user-centered and enables users to have full control over their data. The concept is premised on a combination of technologies, including blockchain, semantic web, immersive technology, and others. The user-friendliness of Web 3.0 is supported, among other things, by granular content distribution.
AI and AI-enabled analytics are among the core building blocks of Web 3.0 as they will help users access relevant data faster. Thus, a website will rely on AI to sift through and provide the data it thinks a specific user will benefit from.
The state of technology today:
Web 3.0 is still in its infancy due to the limited adoption of its technology components. However, as blockchain, cryptocurrency, and connectivity have gathered speed, the hypothesis of Web 3.0 begins to take more shape. Therefore, we can say that some aspects of Web 3.0 have already gone beyond theory.
Metaverse
Metaverse generally refers to an integrated network of virtual worlds accessed through a browser or headset. The technology is powered by a combination of virtual and augmented reality. Unlike Web 3.0, it doesn’t prioritize user ownership over data. Instead, it aims to create a shared digital reality where users can connect, build economies and interact in real time.
The state of technology today:
Currently, Metaverse hasn’t taken its full form yet. The majority of companies are aspiring to develop the Metaverse, including Roblox, Decentraland, Meta, and others. However, those platforms aren’t interoperable. Leading companies are executing metaverse strategies to establish their presence in the existing proto-Metaverse spaces.
Metaverse development trends in 2025 highlight a significant focus on enhancing virtual worlds through AI and blockchain technologies. Key technologies shaping the Metaverse include virtual reality, augmented reality, 3D modeling, and blockchain.
Emerging opportunities are particularly strong in virtual real estate and digital fashion, where innovative applications are driving growth and interest. However, Metaverse development faces several challenges, including issues with technology, cost, privacy, and accessibility.
Edge computing
Edge computing takes cloud data processing to a new level and focuses on delivering services from the edge of the network. This technology allows organizations to process data at the periphery of the network, reducing overall infrastructure costs, improving data sovereignty, and enhancing data security.
The technology will enable faster local AI data analytics and allow smart systems to deliver on performance and keep costs down. Edge computing will also back up autonomous behavior for Internet of Things (IoT) devices.
How the technology is used today:
Industries already incorporate devices with edge computing, including smart speakers, sensors, actuators, and other hardware. These collect data from the real world and process it locally.
Augmented analytics
Powered by ML and natural language technologies, augmented analytics takes an extra step to help companies glean insights from complex data volumes. Augmented analytics also relies on extensive automation capabilities that streamline routine manual tasks across the data analytics lifecycle, reduce the time needed to build ML models, and democratize analytics.
Augmented analytics can lead to better decisions, faster product development, increased profitability, and accelerated knowledge-sharing. The technology also takes data from multiple channels to achieve a broader perspective.
How the technology is used today:
Large-sized organizations often rely on augmented analytics when scaling their analytics program to new users to accelerate the onboarding process. Leading BI suites such as Power BI, Qlik, Tableau, and others have a full range of augmented analytics capabilities.
Engineered decision intelligence
The field of decision intelligence is a new area of AI that combines the scientific method with human judgment to make better decisions. In other words, it’s a way to use machine intelligence to make decisions more effectively and efficiently in complex scenarios.
The goal isn’t just to identify patterns but also to understand why those patterns exist and how they can be used as part of an overall strategy. The technology is supplemented with AI-based capabilities and data fabrics, combined with social science and decision theory.
How the technology is used today:
Decision intelligence assists companies in identifying risks and frauds, improving sales and marketing as well as enhancing supply chains. For example, Mastercard employs the technology to increase approvals for genuine transactions.
Data fabric
Being a holistic data strategy, data fabric leverages people and technology to bridge the knowledge-sharing gap within data estates. Data fabric is based on an integrated architecture for managing information with full and flexible access to data.
The technology also revolves around Big data and AI approaches that help companies establish elastic data management workflows. Data Fabric is usually referred to as an autonomous ecosystem used to maximize access to corporate data rather than a specific platform from a particular software vendor.
How the technology is used today:
Around 26.4% of businesses incorporate data fabrics to enhance business process management. The demand for this architecture is growing in the BFSI sector, retail, E-commerce, and healthcare due to the presence of huge data volumes from multiple sources.
Quantum computing
An antagonist of conventional computing, the quantum approach uses qubits as a basic unit of information to speed up analysis to a scale that traditional computers cannot ever match. The speed of processing translates into potential benefits of analyzing large datasets – faster and at finer levels.
In their commercial stage, quantum computers hold great potential to improve intelligent systems by making them more granular and accurate.
How the technology is used today:
The technology is in its early stage, yet the adoption is spearheaded by increasing funding, proliferating start-ups, and QCaaS offerings. Four industries—pharmaceuticals, chemicals, automotive, and finance—could implement the earliest use cases, according to McKinsey.
Hyperautomation
This concept makes the most of intelligent technologies to help companies achieve end-to-end automation by combining AI-fuelled tools with Robotic Process Automation. Hyperautomation strives to streamline every task executed by business users through ever-evolving automated pathways that learn from data.
Thanks to a powerful duo of artificial intelligence and RPA, the hyperautomated architecture can handle undocumented procedures that depend on unstructured data inputs – something that has never been possible.
How the technology is used today:
Hyperautomation is currently in the ideation state, with classic automation promoting its future growth. Therefore, this trend is now manifested in traditional RPA software that adheres to rule-based tasks and acts on structured data only.
Conversational AI and ChatGPT
In Conversational AI, ChatGPT is a type of chatbot that uses OpenAI’s generative models to create new responses based on the data it is filled with. Having this ability, ChatGPT is more flexible than other chatbots because it can respond to a broad variety of questions so it doesn’t depend on prepared instructions. As a consequence, ChatGPT becomes a turning point in AI development.
How the technology is used today:
The use of ChatGPT is extremely diverse. Its biggest advantage is that it can be used in every business area and boost its revenue a lot. ChatGPT can streamline and automate various work processes, enhance customer service, and improve scalability and speed.
Generative AI
Generative AI is a branch of AI that creates new, original content. Unlike traditional AI, it concentrates on making decisions or recognizing patterns. It depends on deep learning and neural networks to analyze huge amounts of data and use it to create new ones similar to these.
How the technology is used today:
Generative AI can automate, enhance, and streamline various work processes by using enormous amounts of data and generating new content in numerous forms.
Agentic AI
Agentic AI refers to artificial intelligence systems that possess a degree of autonomy or agency in decision-making and actions, often acting independently or with minimal human intervention.
Unlike traditional AI, which typically operates within predefined rules or under direct human control, agentic AI is designed to make decisions, adapt to dynamic environments, and pursue objectives based on its own analysis of the situation. Agentic AI offers several significant benefits across various industries thanks to its autonomy, adaptability, and decision-making capabilities.
Agentic AI’s key advantages
- Increased efficiency and automation
- Enhanced problem-solving and decision-making
- Adaptability in dynamic environments
- Personalization at scale
- Autonomous operation
- Cost reduction
- Scalability
- Reducing human bias.
How to prepare your business for innovation?
Many leaders prioritize short-term analytics gains over a long-term strategy, leading to 85% of data projects failing to meet expectations. Without a solid adoption plan, businesses risk wasted resources and poor ROI. To succeed, organizations must develop a strategic AI adoption roadmap that ensures effective implementation and maximized value.
Here are some milestones that should lay the ground for your pursuit of automation.
Afterword
After the pandemic, the automation craze has passed the tipping point. Today, data analytics and algorithms are an industry standard for high-yielding projects across different verticals. This combination enables global leaders to predict, automate, and optimize processes, reducing time to value. Most importantly, a data-driven strategy fosters integrated business planning, allowing companies to swiftly adapt to new realities.
As we’re stepping into the new era of automation, AI readiness is integral to embracing new technology trends and getting a head start on new initiatives. Be it data fabrics, edge computing, or advanced connectivity, automated workflows, and data control will facilitate your leap to a new level of enterprise success.