The concept of the semantic search was first introduced in already in 1998, but it remained mostly a theoretical concept until 2012 when Google, Facebook and Bing started launching their own products using semantic search.
Two trends pushed semantic search from concept to reality: an increase in long tail searches and a demand from users for more precision.
People were now asking questions in their searches instead of using just a few keywords, and they wanted more accurate results.
Semantic search was (and still is) a good way to address these new demands because it focuses on searches as a whole instead of individual keywords. The context in which search words appear is in the centre of what semantic search is. It is how semantic search engines are able to guess the user’s intent and deliver the most relevant results.
Let’s dive deeper into why semantic search is a superior choice for information retrieval systems, and what benefits it could mean for your business.
Limitations of Keyword-based Searches
The keyword-based approach to information retrieval problems is to build an index, which maps every word to a set of documents where this word is presented.
However, such an approach has a number of limitations:
- The approach offers no benefits in handling lexemes. For example, “child” and “children”, although related, are treated as completely different words.
- Typos are not handled. If the user types “semntic” instead of “semantic” the search will return 0 results.
- Synonyms are not taken into consideration. If a user types “football” the search will not return any documents containing the synonym “soccer”.
This can mean missing out on some business opportunities. For example, InfluencEye, a platform for finding and managing influencers on social media, is using semantic search in their influencer search engine. As illustrated in the example above, the semantic search casts a much wider net and therefore returns a bigger number of potential influencers to work with.
- Generalizations are impossible to understand. If a user is searching for “watersports” he or she will find only documents containing that phrase, but no documents containing “swimming”, “surfing”, “diving” etc.
- All words are treated equally. Connecting words such as “and”, “or”, “if”, etc are given as much importance as more rare words such as “melanoma”, “tensor”, etc.
- Abbreviations cannot be interpreted. If a user types “BTC” he or she is unlikely to find documents containing the word “bitcoin”.
- Homonyms are difficult to place in context. Words that have the same spelling but different meanings make it difficult to return accurate results because we need to look at the context to understand their true meaning.
- Results are not ranked in relevance. Each result from the web or a database is returned only based on the criteria of having the words that the search includes. That is not enough to rank how relevant the results are.
There are, of course, ways to address these issues without semantic search. Lexemes and typos can be approached by performing stemming and lemmatization, checking for misspellings.
Connecting words problem and relevance of the results can be improved by using tfidf vectorization technique and using BM25 for ranking.
The rest of the challenges are, however, not easily addressed without a big commitment of time and resources.
To capture synonyms, generalizations and homonyms you can manually create dictionaries and ontologies (like wordnet), but it requires lots of continuous manual work to create and keep vocabularies and ontologies up-to-date.
That is why you need a semantic search.
What Makes Semantic Search so Powerful
Semantic search has the ability to put searches into context. By looking at the search query as a whole semantic search essentially creates a picture of the user intent, and provide the very best results for the very specific situation of the searcher.
How does semantic search work so intuitively?
To create semantic search engines data scientists use vectors to represent how the meaning of words are related to each other in a certain context. A vector is a quantity determining the position of one point in space relative to another.
This means, for example, that the words “cat”, “dog” and “guinea pig” will be represented with short vectors between each other in the context of a search for “pets”.
The outcome of mapping words and phrases to vectors is called word embeddings.
In other terms, words similar in context, are placed close together in a vector space. Here is a simple example of how it might look like:
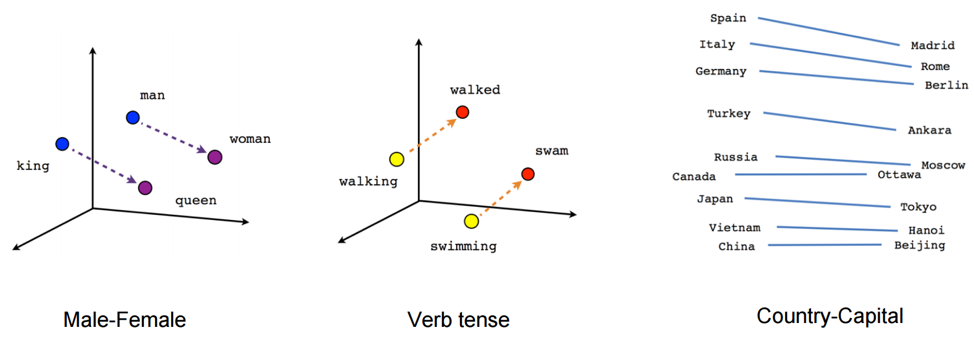
The frequency of the searched words is also taken into the account. This means that words most descriptive of a given search that also appear more often are given a higher score.
Connecting words like “and”, “or”, and “if” appear often but don’t provide any meaning. Their lack of meaning is corrected by inverse term frequency, which accounts for how much information the words provide. That is calculated based on how many times a word appears across all websites or databases.
For example, you search for “what is the biggest mammal”. The descriptive words that would be weighted more are “mammal” and “biggest”. “What” and “is” will be given a lower score.
Popular Approaches to Semantic Search
One of the most popular and, some might claim, the most effective group of models to build a semantic search engine is word2vec. It was developed in 2013 by a team from Google led by Tomas Mikolov.
They proposed several ways of building word embeddings in a vector space. Their solution entailed that every word in a vocabulary has its own dense vector of fixed size. The most useful feature of the works of Mokilov’s team is the fact that words that have similar semantic sense also have similar vectors in that space.
It eliminated the need to manually match similar words to make search engines smarter. You can simply collect a large body of texts and build word embeddings based on the texts.
Benefits of Semantic Search for your Business
Semantic search improves the overall user experience, both before customers land on your site and while they are there, which in turn leads to higher conversion rates.
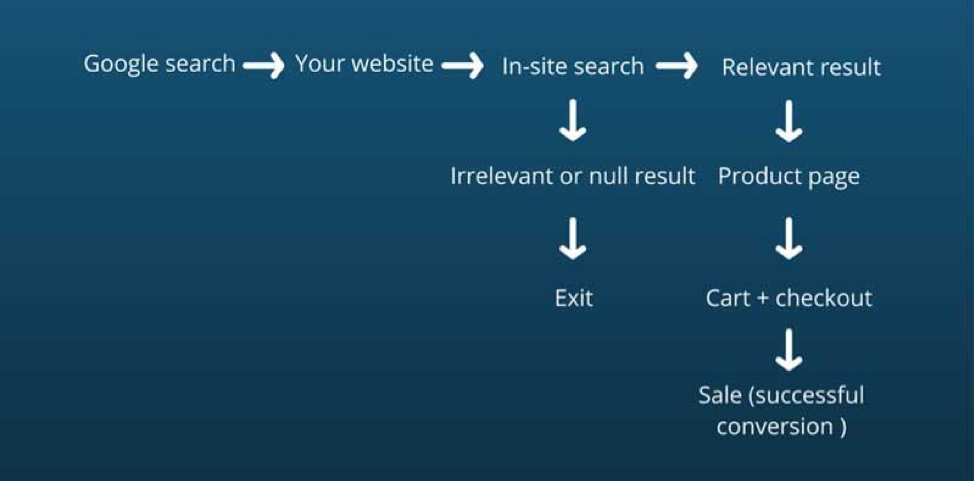
Google is using semantic search to provide more accurate results. This means that Google is looking at the context of your content rather than the presence of the right keywords.
This means your SEO efforts don’t have to be all about the so-called keyword stuffing or optimizing for just a few keywords. This feature allows your marketing team to focus on quality content that covers a topic instead of a single keyword.
Take this example of a search for “where to buy good tea”.
The top search results are tea shops close to where you live. The top organic result that follows does not contain the word “good”. Instead, it has the word “quality”.
Google has understood that when people search for “good tea” they imply quality.
Once potential customers land on your website, semantic search can make a big difference in how your customer can search in your product catalogue. After all, the search functionality of your company’s website is one of the most important tools for conversion.
Semantic search opens up for multiple ways you can customize your search.
- As with Google, you can autocomplete sentences based on popular search and correct spelling mistakes.
- Show product suggestions directly in the search bar
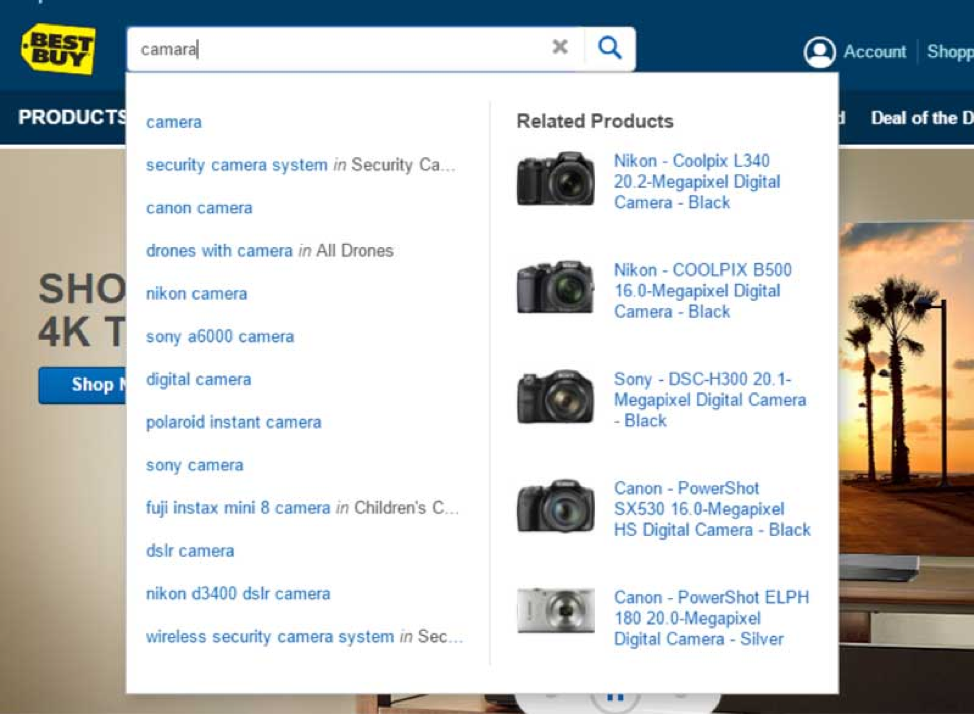
- Semantic search also allows for flexible filters based on what the customer is searching for. Flexible filters act both as a convenience to the customer, and as a way for you to understand what the customer is searching for.
Let’s take a look at how this works.
If you search for “shirt” on asos.com, you get the following filters:
Notice how the option “gender” appears among the filter options. This is because a shirt can be worn by both men and women, and asos.com cannot know your gender (unless you have an account).
Let’s see what happens when we search for “dress”, which is a female attire.
You can see that the filtering options changed to include factors like style, length and dress type.
Semantic Search = More Business Insight
Apart from making the search for products easier for your clients, semantic search can also help you uncover areas of improvement and new business opportunities based on the searches of your customers.
Areas of improvement:
- Most searched terms. Can help you understand the popularity of products, both in terms of specific product groups and brands.
- Common misspellings. This will help you create intelligent auto-suggestions, and uncover any words or product names that are hard to spell.
- Common questions. Especially useful for business that sells services. Semantic search can uncover patterns in the search to identify the most common questions your customers might have while they are on your site.
- Find out which products the customers have trouble finding. Adjust their category or description to increase their visibility.
New business opportunities:
- New product opportunities can be uncovered when evaluating searches for similar products.
- Product pages can be optimized based on frequently used search terms and products the customers buy based on those searches.
- Create sales bundles based on the products the customers usually search for and purchase together.
Conclusion
Semantic search is great for predicting user’s intent when they search. Building a semantic search engine requires many different techniques and models. The business benefits, however, are many.
A semantic search engine can significantly improve the user’s experience on your site. Customers no longer have to worry about typos or using synonyms to find the products they need. Popular products are also much easier to display once a semantic search engine is in place.
Work with InData Labs on Your Next Text Analysis Project
Have a project in mind but need some help implementing it? Drop us a line at info@indatalabs.com, we’d love to discuss how we can work with you.