AI continues to improve every niche that it touches upon. According to the Mckinsey report, Artificial Intelligence (AI) is set to add $13 Trillion to the global economy by 2030 which is about 16% of the total global share. Share on X
In greater detail, AI is a broad term that incorporates everything from image recognition software to robotics. The maturity level of each technology strongly varies. Nevertheless, the number of innovations and breakthroughs that have brought the power and efficiency of AI into various fields including medicine, e-commerce, finance and banking, entertainment and gaming, is only growing. All of the companies undertaking such initiatives had to undergo several changes. And introducing any technological change let alone implementation of artificial intelligence into a company brings a number of challenges.
Though AI is poised to be incorporated by companies in a big way, there are some businesses still hesitant about this new technology. Some fear legal concerns and compliance issues, others face implementation challenges. Have a look at the chart to know more about the main bottlenecks stopping companies from incorporating AI into their businesses.
Source: O’Reilly
Let’s take a closer look at artificial intelligence problems and their solutions.
The Most Common Problems of Artificial Intelligence Implementation
AI Algorithm Bias
Bias is one of the biggest challenges AI has to overcome in the future. Bias can find a way to lurk into algorithms in some ways. AI technology utilizes training datasets to make predictions. And these datasets happen to include human-based decisions laced with gender, sex, race or any other personal information. Back in October 2018, Amazon uncovered that their AI recruiting engine was biased against women. Later on, it was revealed that Amazon’s training models were trained to range candidates by analyzing CVs for a certain period of time. As it turns out those came from men, as they used to dominate the industry. The AI tool devised the CVs containing the words ‘woman’ and ‘women’s’. And there are plenty of stories to back up the theory that for now, AI technology is biased.
Not Everyone Understands What AI Is
To implement AI into business, one should be well aware of its possibilities and limitations, pros and cons. Truth to be told, people barely know what the technology is and how it can address various business challenges. The most popular thing that comes to mind, when one hears the word ‘artificial intelligence’ is robots taking over humankind. The thing is that the lack of understanding of AI technology slows down the adoption of it in many industries. To solve this problem, people should educate themselves on the problem of AI and its current use cases. And maybe slowly but surely, the technology will start paving the way into our lives.
Artificial Intelligence Can Make Mistakes
The idea that AI can be inaccurate or go out of control is quite plausible. There is a chance that AI-driven systems can make mistakes and cause harm if anomalies go undetected. You’ve probably heard about the scenario where AI technology is aimed to go after some virus. In this case, AI is used to study the virus-related genes to create a vaccine for it. Instead of developing a vaccine, AI weaponizes the virus putting humanity at risk.
Take facial recognition technology. The sophisticated technology might oftentimes make mistakes or be inaccurate, if poorly trained. More importantly, when an AI system makes a mistake, it may be really difficult to identify the exact place where something went wrong. Possibly, the best solution might be quality of input, efficient AI software development and powerful bug tracking systems.
Source: Unsplash
AI is Vulnerable to Cyber Attacks
Even the most sophisticated systems have flaws. And AI is no exception to it. Security is paramount in the AI process. It has to be integrated starting from strategic planning throughout the entire cycle. Security is aimed to shield the user’s privacy and their business from data leaks.
Many AI apps are based on the wealth of data. And this data is oftentimes sensitive and personal by nature. The thing is, the AI systems rely on data and cannot go without it. And that is the root of the problem. The more AI trains neural networks, the more vulnerabilities come in the code. The systems get prone to data leaks and identity theft. Even with the GDPR having come into existence, the problem of sensitive data is still unsolved. To avoid data leaks, errors and reputational risks, companies need to put AI security first. The possible way out is to test AI products thoroughly and fix flaws before the products come into the practice or out on the market.
Different Development Approach
Source: Unsplash
AI implementation is quite complicated. Even now, in the era of digitization, it still requires time and effort. Most development in a traditional system environment follows the usual phases such as plan, analyze, design, build, test, and deploy. The AI environment is a bit different. Most of the time, development is about identifying data sources and then gathering content, cleansing it and curating it. Such an approach requires different skills and mindsets, as well as different methodologies. In addition, AI-powered intellectual systems have to be trained in a particular domain.
In case we compare conventional (regular) and AI programming, the differences will look the following way:
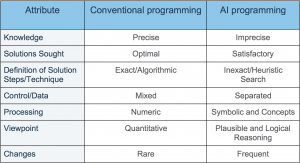
Generally speaking, with AI we are not developing a system but training, giving feedback and supervising an AI-powered solution.
A System is Only as Good as the Data it Learns from
Everyone already knows that AI needs data to learn about things. Specifically, data plays a significant role in the implementation of artificial intelligence. AI and machine learning rely on enormous amounts of high-quality data from which to observe trends and behavior patterns, as well as being able to quickly adapt to improve the accuracy of the conclusions derived from the analysis of that data. Basically, first, you get the data, then you get the AI. The worse data, the more problems you have with artificial intelligence. Such systems don’t just require more information than humans to understand concepts or recognize features, they require hundreds of thousands of times more. Another important thing is the quality of data used to train predictive models. The data sets need to be extremely representative and balanced, otherwise, the system will eventually adopt bias that those
No Clear View on how Insight is Generated
Artificial intelligence advancements are flourishing, as well as its problems. For example, artificial intelligence development is hiding in its experimental nature. It is difficult to say how much of an improvement it may bring to a project. Therefore it is almost impossible to predict ROI. This makes it really hard to get everyone to understand the whole concept. One thing that is necessary to optimize the result is a skilled team that can write or adapt publicly available algorithms, select the right algorithm for the desired result and combine algorithms as needed to optimize the result.
AI is a robust tool poised to revolutionize the world we live in. There’s plenty of innovative solutions AI has brought so far, some are yet to come. Today, AI technology helps a great number of companies meet their needs and stand out from the crowd on the competitive market. But it’s no news that there are benefits and dark sides to every disruptive technology, and AI is no exception to this rule. The important thing for every company is to address challenges and make sure that they can take full advantage of the benefits while minimizing the tradeoffs that may impose artificial intelligence problems.
Let Us Handle Your Problems With Artificial Intelligence Implementation
Schedule an intro call with our AI consulting experts to explore your idea and find out how we can help.