Data science is a very young field that has evolved way beyond simple data analysis. Today, every business wants to increase their data science capabilities, and it’s not surprising why. A data scientist can gather business insights, and develop products that can significantly contribute to the overall business value. Essentially, they make the data more understandable and useful to the rest of the company.
The whole data science process is, however, long and complicated. Before anything else, the data needs to be collected and cleaned from any irrelevant data entry points. Data preparation is considered to be the most time-consuming part of the process because companies tend to accumulate big amounts of data stored in multiple formats and at different places, making it harder to convert all this data into one usable format. After the data is clean, it needs to be modeled according to the needs of the company or the project in order to finally extract valuable insights from the data.
Once the modeling is complete, the insights need to be conveyed to the rest of the company. Essentially, the results of data modeling need to be visualized in order to provide any value. Often, that requires a completely different skill set that has little to do with data science and more with presentation and communication skills. At this stage, the company has an option to act based on the results, or develop a product that will, for example, improve customer experience and bring value to the company.
Why you Need a Fully Integrated Data Science Team
All the steps mentioned above require different knowledge, background, and skill set. In addition, the complexity of the whole data science process varies depending on what kind of data your company is generating. For example, working with images and sounds calls for different data processing skills compared to marketing or analytics data. Getting good at one step of the process or one type of data is difficult, let alone all of them.
Use this grid to help you think about the talent you need to hire:
The demand for data scientists is growing much faster than education institutions can meet. The following summary graphic from IBM’s job market research highlights how in-demand data science and analytics skill sets are today and are projected to be through 2020.
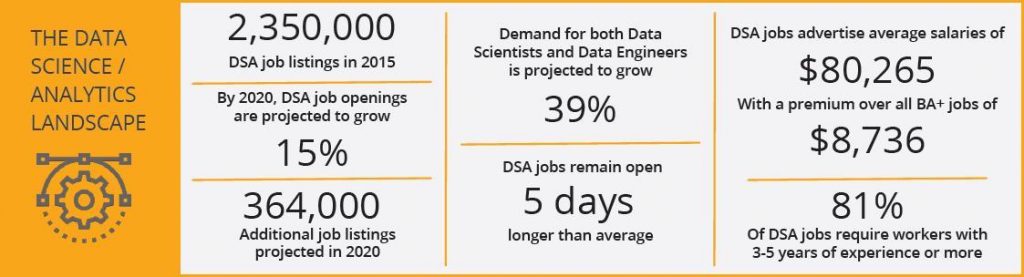
Glassdoor ranked Data Scientist as the number one best job in America in 2017 due to its high job satisfaction, high demand, and generous base salaries. Many people from different technical disciplines are migrating to the field of data science because of that. Some of them come from science and math backgrounds, while others from computer science or statistics. None of them are better in excelling at data science than the others. It just means that the candidates will have different strengths and weaknesses that make them better or worse at some steps of the data science process. As an employer, you need to be aware of that, and look for candidates that have the strengths that are needed for your type of data. The team makes all the difference between a successful and a failed data science project.
The bottom line is, each step should ideally be executed by one person with experience and capabilities in that particular area. That means that the ultimate solution is to have a whole team of data scientists. The team should at least consist of a data engineer, a machine learning expert, and a business analyst. Data engineers are responsible for data collection, data warehousing, data transformation, and data analysis. This role should be filed by an analytical person with a background in tech. A machine learning expert is a link between data engineers and business analysts. They build predictive models, develop algorithms and make sure that the derived data is accurate and easy to understand. Business analysts do some data analysis as well, but their main responsibilities are data exploration and data visualization. They are responsible for connecting the insights derived from the data to the overall business objectives.
Building up Data Science Capabilities
1. Hiring a Data Scientist
Hiring a data science team can be very expensive, but having data scientists in-house can be beneficial for multiple reasons. First of all, they will know your data better because they will have the possibility to fully emerge themselves in it over longer periods of time. Secondly, they will be your employees, meaning they will make more effort to fully understand company goals, and ultimately care about the product or service that you offer. That is something that is hard to achieve when working with consultancy firms.
On the other hand, as we discussed before, it’s hard to find good data scientists, and hiring bad ones can be very costly. For example, the data can be modeled in the wrong way and bring no particular business value. That means that all the money invested in the data science project will be wasted.
Hiring and training data scientists represent a significant part of the total costs of a data science project. First of all, while you are searching for people with the right skill set, you are losing time during which your company could be developing a new feature or product.
Secondly, training data scientists is costly. There are a number of good programs that help train data scientists, but the best way to become a data scientist isn’t to be trained but to do real work. For that reason, either you have to invest in a robust infrastructure in order to do some serious, data-intensive work, or you can hire talented graduates and invest in a good internship program.
2. Hiring a Data Science Consulting Company
It can be beneficial to partner with a data science consulting company that has the knowledge and experience to help you get the full value out of your data science project. A data science consultancy company is essentially a powerhouse that specializes in one thing only. That means that they invest heavily to have skilled staff that has expertise not only in the field of data science but also design skills and specific domain expertise. You won’t have to search or train your own data science team, meaning hiring a consultancy can actually save you time and money in the long run.
If you decide to go the consultancy route, it can be beneficial to choose a smaller consultancy company to partner with. They tend to price themselves lower than their bigger, corporate counterparts. Smaller boutique consultancy firms tend to have less overhead cost and payroll expenses. Their office space needs are typically smaller and they attract talent with interesting projects rather than six-figure salaries. More importantly, smaller businesses have less bureaucracy, and fewer projects to work on simultaneously. Therefore, you are more likely to get better service in terms of higher engagement in your business challenges and faster delivery times.
Wondering how to go on about choosing the right data science consultancy for your business? Stay tuned for our next article.
Work with us on Your Next Data Science Project
Schedule an intro consultation with our Data Science Experts to explore your idea and find out if we can help.