Artificial intelligence has made significant inroads into the domain of pharmacological research. AI in drug development is also a hotly debated topic due to its contribution to RWE analytics and candidate drug identification. But while experts are bickering over its potential in the field, the global market of AI in drug development follows a growth trajectory. By 2030, the market is set to reach $9.1 billion.
Today, we’ll have a close look at the synergy of AI and drug development, including the headline-grabbing RWE analytics.
The fundamentals of AI in drug development
According to research (nature.com), AI drug development companies have over 150 small-molecule drugs in discovery and more than 15 already in clinical trials. This huge achievement wouldn’t be possible without intelligent systems.
AI in drug development is a process where automation is applied in the research and development of new drugs. It involves the use of machines to learn how new drugs work and to predict how they will behave in the human body. This helps to speed up the drug development process, as well as improve the accuracy of predictions.
Moreover, data science and AI companies make such waves in the pharmaceutical industry thanks to data processing capabilities. Machine learning algorithms can learn from large amounts of data quickly and make accurate predictions with a high degree of certainty. It means that computers can sift through an ever-growing amount of data to identify promising candidate drugs.
Benefits of AI drug development
The pharmaceutical sector has had it hard with an overflowing amount of data. The digitalization the sector is experiencing inevitably incurs challenges. Data processing, scrutinizing, and analysis are utterly important, yet difficult for drug researchers.
Below, we’ve listed the main benefits of artificial intelligence for healthcare in general, focusing on drug development.
Speed of analysis
Evotec, a German biotechnology firm, launched a clinical trial on a new anticancer drug in 2021. The candidate discovery procedure took eight months, despite the fact that it sounds routine. Artificial intelligence algorithms were directly responsible for this unrivaled speed.
Sophisticated systems can sift through millions of potential tiny molecules and compare their many features automatically. It means that the analysis process is majorly governed by AI, thus reducing the development and analysis time.
Predictive potential
Predictive analytics and the pharmaceutical industry are a powerful combination that can lead to better patient outcomes. Thus, artificial intelligence can be used to predict ADME properties as well as effectiveness and safety issues. Of 10,000 small molecules initially screened, only 10 are selected for clinical trials.
Intelligent systems can reduce the time needed to analyze them by predicting their effectiveness. Moreover, AI can alleviate one of the biggest bottlenecks in drug development: the high failure rate of experimental drugs late in the process.
Cost savings
The average cost of the R&D process equals $2.168 billion per drug. The lion’s share of this investment is spent on the drug discovery phase. AI-based drug development can reduce the resources needed to screen and synthesize the drug by as much as $26 billion annually.
Cutting-edge drug discovery algorithms
Machine learning solutions providers usually stay at the edge of innovation to introduce the latest technology into the algorithms. Combined with robust processing power, AI for drug development presents a unique mix of health data and technology. This combination, in turn, complements the benefits mentioned above.
Better success rates
According to the statistics, 90% of drug candidates fail during the 1st phase of clinical trials. If we factor in the failure rate at the preclinical stages, this percentage will look even more daunting. Intelligent algorithms can revert the numbers by assessing biomarkers that reflect the effectiveness of the medication.
Artificial intelligence and Big data for healthcare also establish a single blanket of data. Fuelled by the large amounts of medical data, advanced machine-learning algorithms can assess the whole variety of insights, which contributes to the disease markup. All combined, researchers will have a better view of the disease and effective drug candidates in each specific case.
AI for drug development: the application matrix
A typical drug discovery cycle, according to the FDA, has five steps. All of it begins with laboratory research. The candidate is then put through a series of tests before being put into clinical trials and human testing. The FDA next evaluates the drug and decides whether to approve or reject it. FDA also monitors every medicine and device safety once they are released.
AI drug development companies can introduce machine intelligence in the first three stages. It helps increase the speed and reduce the costs of research and discovery. Below, we’ve curated the main applications of AI in drug development by stage.
Stage 1: Drug Discovery
The most crucial component of the drug discovery process is the early stages. During this stage, possible applicants are screened. High throughput screening (HTS) is the most popular screening tool used in the early stages of drug discovery. It incorporates the use of robotics and automation to swiftly evaluate huge numbers of compounds.
Here’s how researchers identify a compound that can improve patient outcomes:
And here’s when artificial intelligence steps in. Artificial intelligence can help scientists go through the millions of compounds in their databases faster, more efficiently and with greater accuracy than human scientists alone can achieve.
This means that fewer compounds need to be synthesized in order to find those that have therapeutic properties. Let’s have a closer look at how machine intelligence amplifies the first stage of development.
Target protein structure prediction
Predicting protein structure is a revolutionary approach in healthcare. With a protein structure on hand, researchers can develop a better understanding of how a protein works. This, in turn, can help researchers generate hypotheses about how to affect it. However, a 3-D protein structure must be in sharp focus to benefit the discovery. It means that each atom’s position should be discovered.
This area has benefited significantly from the application of artificial intelligence, which is used for target protein structure prediction. Protein folds are 3D structures that give proteins their unique functional characteristics. The majority of proteins in a biological system fold into a small number of common architectures. In order to understand how a particular protein functions, it is important to know its fold.
The use of artificial intelligence for target protein structure prediction is a powerful tool that can be used to improve our understanding of the three-dimensional structures of proteins.
By using machine learning methods, we can develop computer models that are capable of accurately predicting the structures of proteins. This approach has already been used to great effect in many cases and is likely to play an increasingly important role in drug discovery and other aspects of biomedical research in the future.
For example, Deep learning algorithms, including AlphaFold2 and RoseTTAFold, can now help predict a protein’s 3D shape from its linear sequence. The AlphaFold2 algorithm is courtesy of Google’s DeepMind’s artificial intelligence system. The tool is readily available to the public and generates a prediction in one click.
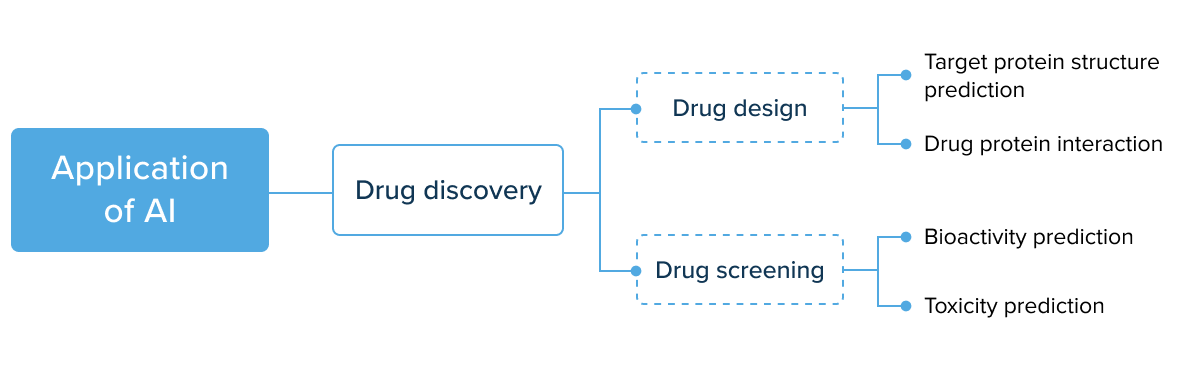
Compound screening
The screening and development of new drugs is a process that requires the analysis of billions of data points. AI drug development can help researchers to more accurately identify lead molecules, which can accelerate virtual screening hundreds of folds. In addition, machine intelligence can also create predictive models for drug toxicity.
According to research, intelligent software can be used to predict the binding affinity of a ligand to a receptor, as well as the rate of dissociation. This knowledge allows researchers to assess the intermolecular interactions induced by medicine. In addition, AI can also be used to design novel ligands that have improved binding affinity and selectivity.
It’s data science and artificial intelligence that brought together leading pharma companies in 2020. In their pursuit of a COVID-19 vaccine discovery, Pfizer, Amgen, Takeda, and other leaders teamed up to develop and gain regulatory approval faster and with no lags. Artificial intelligence is also used for compound screening at Schrödinger, Atomwise, and others.
Finally, AI for drug development is uniquely positioned to support iterative screening. The latter is a much-awaited enabler of innovative high-throughput screening or HTS. HTS is commonly employed to find HITs – compounds with confirmed activity against a target. Thanks to machine learning, iterative screening can better describe the biology and produce higher return rates.
Stage 2: Clinical trial design and monitoring
Clinical trials form the foundation of drug discovery and development. By providing a rigorous scientific evaluation of the safety and effectiveness of new treatments, they allow us to determine whether a potential medicine is ready for use in patients. Historically, this process has been accomplished through a labor-intensive and expensive endeavor, requiring the participation of many people.
Clinical trials are conducted in phases in order to test the safety and effectiveness of a potential drug. Clinical trial data is then routinely cleaned and analyzed by statisticians to determine whether the drug should be progressed to the next phase or not.
At the time, ClinicalTrials.gov lists over 418,021 studies. However, the majority of them struggle to enroll enough people for comprehensive results. In recent years, however, artificial intelligence has been used to speed up and improve the accuracy of clinical trial selection.
Subject and site selection
Recruiting the right patient cohort plays a crucial role in the overall success of the trial. Researchers are to enroll the patients who will be most likely to benefit from the treatment being studied. AI-based healthcare IT solutions now support patient recruitment and site identification to perform trials.
Source: Deloitte analysis
In particular, researchers employ AI and ML algorithms to find the sites with the highest recruitment pool and suggest recruitment strategies. From population mapping to predicting patient potential, artificial intelligence and data analytics support the entire selection process.
Automated systems also analyze patient records and real-world evidence to match patients with trials. The IQVIA company leveraged the ML-based recommendation to increase the enrollment rate to 20.6% compared with the traditional experience-based site identification approach.
Patient dropout
Around 30% of patients drop out during a clinical trial. At the same time, 40% are non-adherent after 5 months, which incurs additional costs for the pharma industry. High dropout rates are caused by the overwhelming amount of reporting to be made by patients. Medication intake, bodily functions, and other response data have to be logged in on a daily basis.
Source: Unsplash
Artificial intelligence, however, is making strides in increasing patient retention and medication adherence. Combined with wearables, algorithms can automatically read and process patient data.
Deep learning models can then process this data in real-time and transfer the insights to clinical trial investigators. Predictive analytics providers can also deliver intelligent models for predicting the risk of dropouts.
Clinical trial analysis
During the study closeout, researchers have to deal with immense volumes of operational data. However, data silos and isolated data infrastructure hide the lion’s share of valuable insights. In particular, a global trial portfolio is the most challenging to put together. Scattered geo-based data is siloed on local servers and cannot be put fast for analysis.
AI-enabled analytics platforms can aggregate all data and vital metrics in one place. Powered by algorithms, trial data is cleaned and analyzed, while reporting tools visualize the insights for further decision-making.
Once the new drug has been tested and developed for maximum efficacy and safety, it is sent to the FDA for a comprehensive assessment. The FDA evaluates the findings of clinical studies and approves or rejects the medication application.
RWE analytics
RWE analytics is the study of the real-world effectiveness and value of pharmaceuticals and medical treatments. This phenomenon holds a special place in the pharma industry due to its untapped potential.
This massive body of knowledge acts as a blanket of healthcare data that can then be employed for a variety of analytical tasks in medicine. RWE analytics can also offer new research paradigms for precision medicine.
Advanced analytics in RWE relies on large data sets that include patient outcomes gathered from insurance claims, electronic health records, and other sources.
This data is then used to assess not just a drug’s clinical benefits but its overall value to society. Real-world evidence data analytics is seen as an important tool in the drug development process, helping to ensure that therapies are both clinically effective and cost-effective.
Real-world evidence strategy and analytics also yield vital knowledge on different patient populations and their characteristics. For example, in March, a CDC study used RWE analytics to assess the effectiveness of mRNA COVID-19 vaccines.
However, the generation of real-world evidence requires lots of processing power to transform raw data into knowledge. That’s when artificial intelligence steps in.
Real-world evidence analytics
According to McKinsey, real-world evidence analytics can help pharma companies unlock over $300 million a year. And machine learning is among the main pillars of RWE adoption.
Intelligent algorithms can help generate critical information to address issues that would otherwise be too costly and time-consuming to solve.
In particular, machine learning presents unprecedented opportunities to combine data from different sources into a single source of truth. Algorithms can then tap into the integrated data environments and run analytics models continuously and at scale.
But where do real-world evidence strategy and analytics fit into drug discovery? The output of RWE is present at all pharmaceutical stages – from research to clinical development. Due to time and resource constraints, researchers aren’t able to study every possible combination of therapy. Combined with automation, real-world evidence data analytics takes over the hard job.
According to the FDA, the agency relies on RWE to keep tabs on post-market safety and support regulatory decision-making. Healthcare providers lean on real-world evidence strategy and analytics to power coverage decisions and health support tools for use in clinical practice.
RWE insights also lay the ground for clinical trial designs and studies to create innovative, data-driven treatment approaches.
Challenges of AI drug development
The ability of AI systems to rapidly generate hypotheses for drug targets is a major advantage over conventional approaches. This allows scientists to explore many more possible treatments and quickly identify promising candidates for further research. However, AI-developed drugs are still in their nascent stage due to a number of challenges.
Data collection
Intelligent systems live off massive datasets to produce a high accuracy rate. Therefore, availability and quality of data are two cornerstones for AI adoption in drug development. However, the healthcare industry is one of the slowest AI adopters. Data access issues, regulatory problems, and misaligned intentions are stated as the main inhibitors.
Moreover, rare diseases present a small sample of studies, thus lacking enough analytical data. This makes it difficult for researchers to collect enough data points from which they can accurately train an AI algorithm.
Source: Unsplash
Data analysis
While predictive analytics for businesses processes somewhat straightforward data, patients’ genetic makeup presents an analytical challenge. Biological characteristics and treatment history interact in complex ways. This, in turn, results in unpredictable drug responses. The outcome is hard to analyze and predict.
Thus, pairwise drug-dose combination responses are easily predicted by ML models. Yet, higher-order combination effects with over two drugs are still difficult to assess. Therefore, the accuracy of ML algorithms is still far from wide application in pharma.
Algorithm transparency
Being the most robust technology, deep learning is the black box of artificial intelligence. Although accurate, deep learning prediction is elusive to the human expert. Its non-deterministic nature doesn’t presuppose straightforward processing. Instead, researchers can see the output with no interpretation.
For example, IBM’s engineers highlight that smart systems must comply with the four pillars of trust. Fairness, explainability, robustness, and transparency should be put at the center of all algorithms.
Until the computer’s rationale is hidden, researchers cannot fully rely on algorithms to produce critical decisions.
Google, for example, took a hand in making AI more interpretable. Its Explainable AI offers a sneak peek into the inner workings of machine learning models.
Data ownership
Patient datasets are another challenge that backpedals a unified data analytics pool. Although healthcare institutions collect patient data, they cannot be considered its owners. Instead, they serve as data custodians and cannot share any sensitive data with each other.
Each data piece is the property of the patient. Data sharing can only be permitted through patient consent. Therefore, most data sets are proprietary and cannot be sent for analysis and research purposes.
The final word
The development of new drugs is a complicated process. It takes many years to develop a drug from the initial idea to its approval by the FDA, and then another eight years on average before it reaches the market.
But AI has the potential to drastically reduce this time frame while minimizing costs and improving the accuracy of drug discovery.
At the same time, AI technologies can help researchers predict how molecules will interact with each other, which helps them avoid creating drugs that are toxic or cause off-target effects on humans.
Despite present challenges, AI-developed drugs are slowly making their way into the pharma industry. Due to the data growth, we’ll likely see the untapped potential of AI go into full gear in the coming years.
Explore drug Development with AI
Looking for a reliable technology partner to help with AI-driven drug development? Hit the link to schedule a call, and our specialists get back to you soon.